One of the fundamental goals of SIMCor is to validate the safety, efficacy and usability of cardiovascular implantable devices for patients through the use of in-silico-based methods, which include the virtual implantation and the effect simulation of medical devices in virtual patients.
In SIMCor, our approach is validated on two clinical use cases of relevance, heart valve prostheses implanted via transcatheter aortic valve implantation (TAVI) procedure, a common, highly-investigated procedure for the treatment of aortic valve disease, and a pulmonary artery pressure sensor (PAPS), developed by our partner Biotronik (BIO) for the monitoring of heart failure patients.
This approach has its limitations. The properties we investigate through modelling and simulation approaches, the so-called “in-silico clinical trials”, are related to the performance and adverse effects related to mechanical properties of such devices, and are thus defined as “engineering metrics”.
When we assess the robustness, utility and safety of a medical device to be used in patients, though, what we are interested in are the clinical outcomes of such procedures. The last step for closing the loop is thus linking engineering metrics with clinical outcomes for patients.
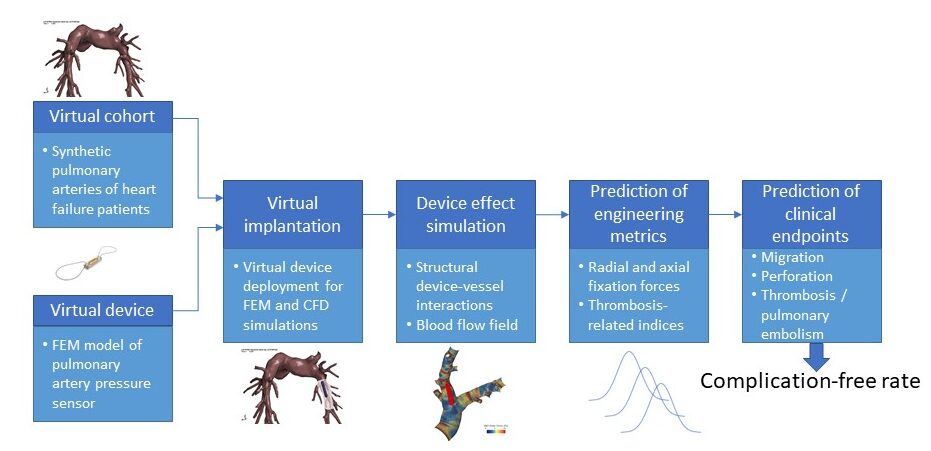
SIMCor, as a consortium, has been posing this issue and has tried to address it by formulating a draft strategy for the mapping of our engineering metrics under investigation to the relevant clinical outcomes of interest. This is based on a chain of transfer functions linking the engineering metrics obtained in an in-silico trial with the clinical outcomes investigated in a relevant patient trial. This strategy relies on a few key steps.
- Assign engineering metrics to clinical outcomes: this consists of defining numerical model output variables, corresponding to clinical risks and outcomes of interest in patients.
- Match model predictions with (pre)clinical data:
- Match model prediction of known device with clinical data: when a device, such in the case of TAVI, is already on the market, and we have an improved version to validate, we can rely on medical literature related to medical device trials to connect the reported ranges of engineering metrics for those devices to the short, medium and long-term effects reported in patients.
- Match engineering metrics with pre-clinical data: for novel devices where no clinical data is available, step 2a is not possible. In this case, we can rely on preliminary animal studies, such as chronic and acute studies assessing device implantation, to derive a set of candidate clinical outcomes, to be verified later on in dedicated clinical studies.
- Derivation of acceptance criteria and model calibration: in either ways, these data can allow to derive acceptance criteria for the engineering metrics of the target devices to serve as indicators of clinical acceptance, and serve as guidance for calibration of the new/improved device.

This work, initiated by our consortium but still under development, has now been expanded to an inter-project collaboration with other relevant consortia working on in-silico cardiology, such as SimInSitu and SimCardioTest, into a multidisciplinary working group.
Among other open issues in in-silico cardiology, this topic will be the object of a dedicated working group of an inter-project cardiovascular modelling workshop, to be organised by the in-silico cardiology consortia, under the lead of SimInSitu and SIMCor, for the beginning of 2024.